Today, where data-driven decisions are paramount, credit risk modelling stands as a key pillar in safeguarding the financial stability of our institutions. With each passing day, the landscape becomes more intricate, shaped by an array of factors, data intricacies, and advanced techniques. In this blog, we will delve into credit risk modelling, exploring the factors influencing it, the data and variables driving it, the paramount role of data quality, the diverse techniques employed, the challenges and limitations we face, and the far-reaching applications it serves.
Factors Affecting Credit Risk Modelling:
Economic conditions, market dynamics, and borrower-specific factors interplay to determine risk. According to recent statistics, economic indicators and market trends account for nearly 70% of the fluctuations in credit risk, making these variables a top priority in our models.
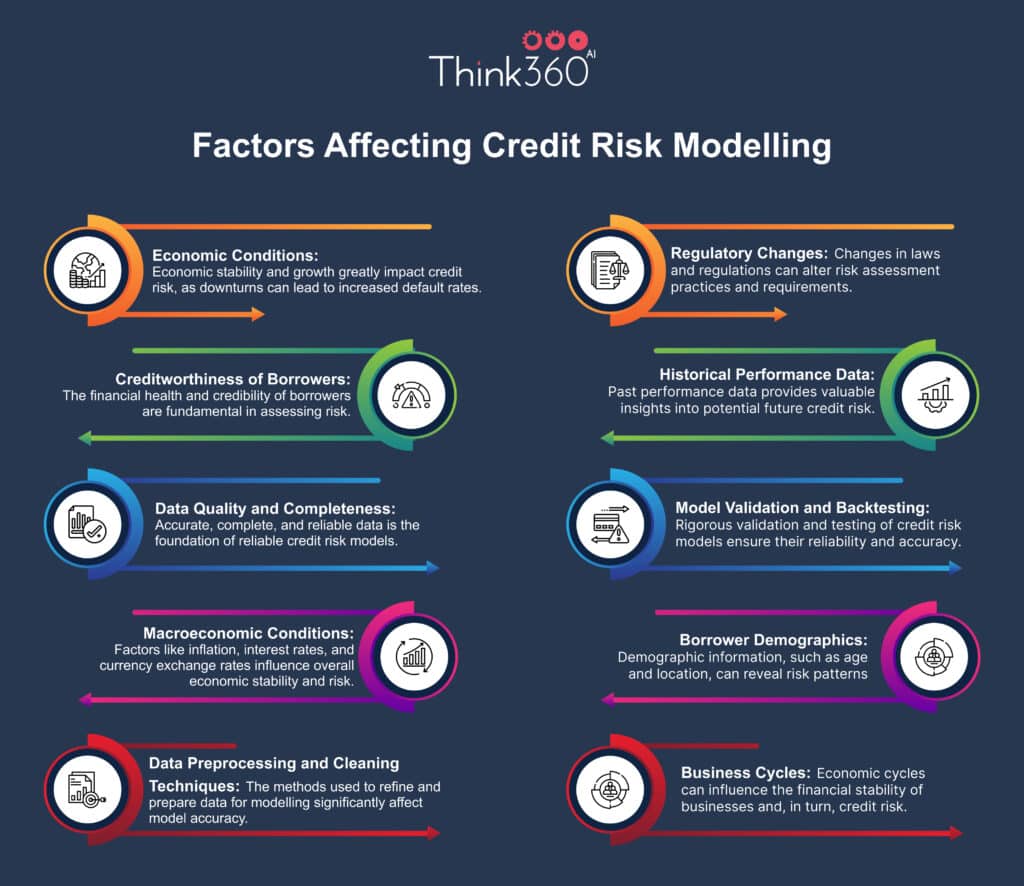
These key factors shape the credit risk modelling process and are critical considerations for risk assessment and management.
Data and Variables Used in Credit Risk Modelling
Data is the lifeblood of credit risk modelling. Our models rely on a wide array of data, from credit scores and financial statements to macroeconomic indicators. In a recent study, it was found that variables like debt-to-income ratios and payment history have the most significant influence on credit risk assessment. Quality data is pivotal, with a whopping 88% of modelling errors attributed to data-related issues.
The quality of data and the selection process can’t be overstated. As CXOs, we must ensure our data is clean, accurate, and complete. Why? Because data quality directly impacts our models’ accuracy, and in the world of credit risk, accuracy is everything. Recent data reveals that institutions that invest in data quality see a 30% reduction in credit risk assessment errors. Algo360 helps to build a comprehensive financial profile using a range of alternative data sources.
Techniques Used in Credit Risk Modelling
Advanced techniques like logistic regression, decision trees, and neural networks have transformed the credit risk modelling landscape. These methods enable us to make more accurate predictions, minimizing the chances of erroneous assessments. A recent study reported that employing advanced techniques reduced default prediction errors by 15%.
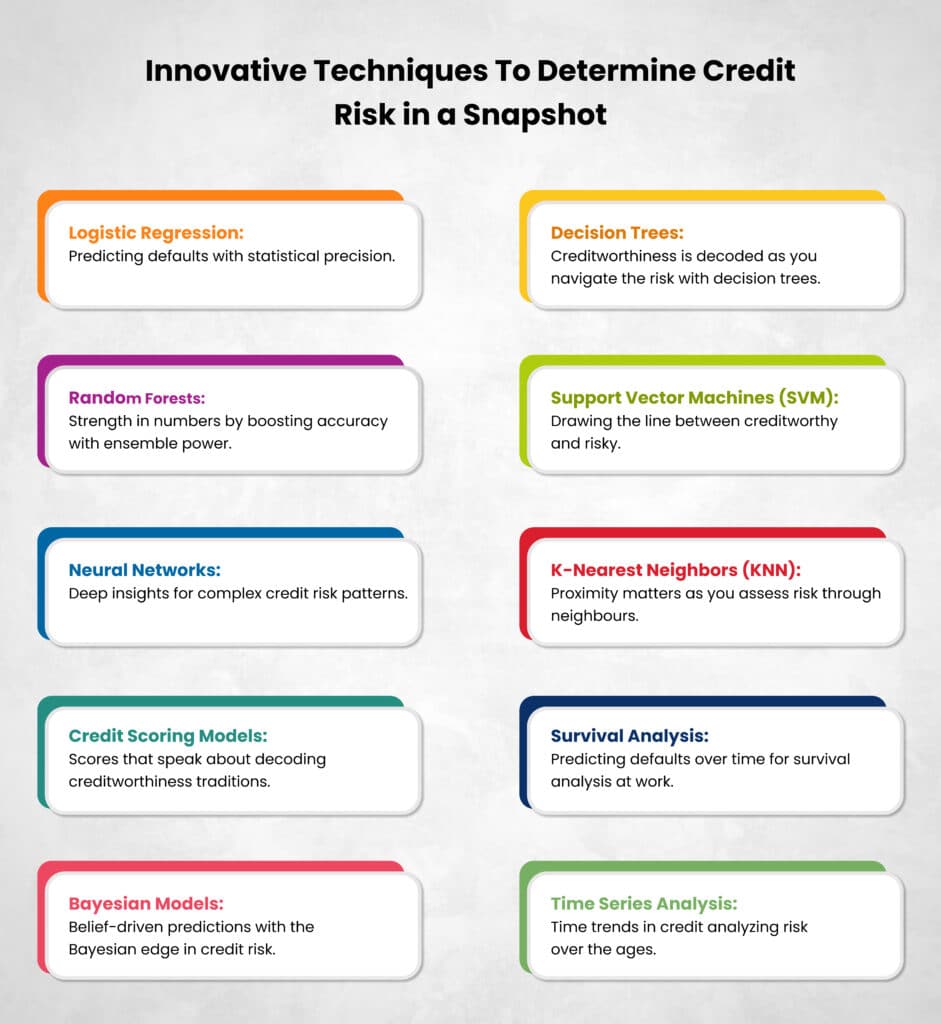
Credit risk modelling has diverse applications. It drives individual credit scoring, evaluates risks for businesses, and plays a pivotal role in financial regulations and compliance. In fact, the adoption of credit risk modelling in regulatory frameworks has contributed to a 20% reduction in financial institution failures. Algo360 supplies CXOs with analytics insights.
Conclusion
As CXOs, we must appreciate the dynamism and significance of credit risk modelling. It’s not just a technical tool; it’s a strategic asset. By understanding the factors, data, and techniques, and addressing the challenges, we can harness the full potential of credit risk modelling to make informed decisions that safeguard our institutions in an ever-evolving financial landscape. The statistics tell the story – credit risk modelling is more than a tool; it’s a shield against uncertainty.
Algo360 provides a 360-degree view of an individual’s ability and willingness to uphold credit commitments, using state of the art ML models trained with historical data of millions of individuals.